Auditing Proprietary Algorithms while Preserving Privacy is Possible: Here's How
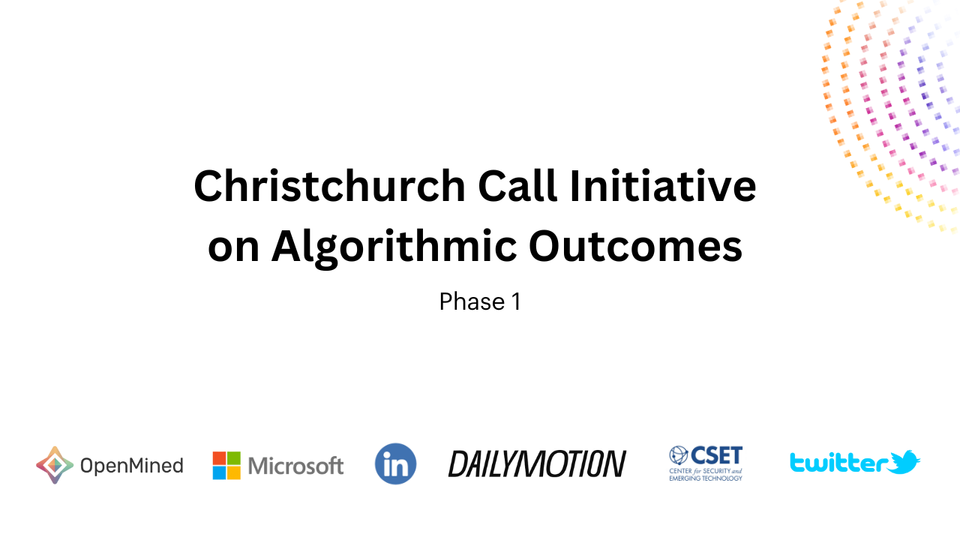
Written by Dr Nicole Matejic
When the Christchurch terrorist livestreamed his attack on 15 March 2019, the footage quickly went viral across social media. It didn’t go viral because people actively sought it out, instead, it appeared in people’s feeds because social networks did exactly what they were programmed to do – recommend content that their algorithms rank as relevant to their users. As more people discovered the footage in their feeds and expressed their shock, outrage and horror, algorithms responded by amplifying it further. The result was millions of people becoming unwilling witnesses to these horrific acts of terrorism.
Recognising the inherent harms in the way algorithms treat content out of context, not only in amplifying terrorist and violent extremist content (TVEC) but also contributing to online radicalisation, the 2022 New Zealand/France Christchurch Call Leaders' Summit Joint Statement endorsed further work to examine algorithms. Then Prime Minister of New Zealand and now Patron of the Christchurch Call Foundation Dame Jacinda Ardern announced the Christchurch Call Initiative on Algorithmic Outcomes (CCIAO).
Today, the Christchurch Call together with its CCIAO partners OpenMined, Microsoft, LinkedIn and Daily Motion published a Phase 1 report demonstrating the viability of using PySyft to facilitate algorithmic auditing by external researchers.
Third-party algorithmic audits are crucial for AI transparency. They enable the identification of risks such as unfair bias, intellectual property violations, the spread of disinformation and/or other harmful content types.
As part of CCCIAO’s Phase 1 project, four independent researchers successfully performed audits of recommender systems at LinkedIn and Dailymotion, whilst protecting the security and privacy of personal and commercially sensitive information through the combined application of remote data access and differential privacy. These privacy guarantees, alongside the governance processes built into PySyft, ensured that both LinkedIn and Dailymotion had sufficient confidence in making real, anonymised, production data available to researchers.
In other domains, findings from third-party audits have led to critical interventions including voluntary moratoria or regulatory changes. However, it can be prohibitively challenging to conduct third-party audits of AI systems due to legitimate security, privacy, intellectual property, or trade secret concerns, which prevent external auditors from being able to access and study the key data assets that are consumed or produced by the AI system.
To achieve proof of concept, those working on the CCIAO Phase 1 project had to overcome those challenges. Traditional audits of a proprietary system require that the auditor (a) obtains a copy of the data/model/software, (b) goes on-site to have direct access, and/or (c) uses an API the company created. Whilst these approaches can be successful, they are not without limitations. CCIAO Phase 1 aimed to overcome the limitations of these approaches by using new oversight tools that enable privacy-preserving audits. The purpose of Phase 1 was to build audit infrastructure that leverages these tools, that independent external researchers could then test to assess their feasibility.
Enter PySyft, an open-source library that implements a flexible approach based on remote data science. One of the benefits of PySyft is that data owners decide how and under what conditions third parties can remotely access their relevant datasets. This approach allowed the project to integrate other third-party privacy-preserving tools, such as differential privacy, which helps ensure that the outputs of queries preserve user anonymity. Additionally, remote data science and differential privacy add additional layers of security and privacy, allowing third parties to study proprietary systems without direct access, thus mitigating the largest concerns about privacy and security.
Like all research, the study has limitations. Domain specific knowledge is required by the research team. Context remains key to any robust analysis. The sample size and type of data studied was limited and would benefit from access to a broader array of networks. Similarly, there are unresolved questions around how the evaluation of algorithmic results against the real-world impacts of radicalisation and TVEC can be achieved. These are challenges shared globally among those working to prevent radicalisation to violent extremism and, separately, to better evaluate information integrity issues.
We continue to explore opportunities for the Call Community to support and lean into these efforts as we know there is more work to be done. Phase 1 is the first of 4-5 phases of work that need to be completed to realise the vision Call Leaders had in 2022 of delivering tools at scale to accredited researchers, with the support of technology companies in the Call community.
As we now move into Phase 2 of the CCIAO, we’ll be building on this important work as we look to foster this new field of algorithmic research and share what we learn.
Download the full report here:
Dr Nicole Matejic is a Principal Strategic Advisor with the Christchurch Call Foundation.